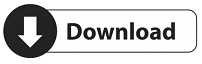
It involves predicting the movement of a person based on sensor data and traditionally involves deep domain expertise and methods from signal processing to correctly engineer features from the raw data in order to fit a machine learning model.
#HUMAN ACTIVITY DETECTION MATLAB CODE SERIES#
Mucha, Peter J.Human activity recognition, or HAR, is a challenging time series classification task. Zhong, Linfeng Xue, Xiaoyu Bai, Yu Huang, Jin Cheng, Qing Huang, Longyang Pan, Weijun: Information spreading on activity-driven temporal networks with two-step memory (2021).Bedru, Hayat Dino Yu, Shuo Xiao, Xinru Zhang, Da Wan, Liangtian Guo, He Xia, Feng: Big networks: a survey (2020).Hsu, Bay-Yuan Tu, Chia-Lin Chang, Ming-Yi Shen, Chih-Ya: CrawISN: community-aware data acquisition with maximum willingness in online social networks (2020).Interdonato, Roberto Magnani, Matteo Perna, Diego Tagarelli, Andrea Vega, Davide: Multilayer network simplification: approaches, models and methods (2020).Meeks, Kitty Skerman, Fiona: The parameterised complexity of computing the maximum modularity of a graph (2020).Mihm, Maximilian Toth, Russell: Cooperative networks with robust private monitoring (2020).Paul, Subhadeep Chen, Yuguo: Spectral and matrix factorization methods for consistent community detection in multi-layer networks (2020).Paul, Subhadeep Chen, Yuguo: A random effects stochastic block model for joint community detection in multiple networks with applications to neuroimaging (2020).Ghosh, Dibakar: Intralayer synchronization in evolving multiplex hypernetworks: analytical approach (2020) Sun, Chengbin Luo, Chao: Co-evolution of influence-based preferential selection and limited resource with multi-games on interdependent networks (2020).
Vaiana, Michael Muldoon, Sarah Feldt: Multilayer brain networks (2020). Wang, Dingjie Yu, Wei Zou, Xiufen: Tensor-based mathematical framework and new centralities for temporal multilayer networks (2020). Rasti, Saeid Vogiatzis, Chrysafis: A survey of computational methods in protein-protein interaction networks (2019).įonoberova, Maria Sahai, Tuhin: Spectral complexity of directed graphs and application to structural decomposition (2019). Xia, Chengyi Wang, Zhishuang Zheng, Chunyuan Guo, Quantong Shi, Yongtang Dehmer, Matthias Chen, Zengqiang: A new coupled disease-awareness spreading model with mass media on multiplex networks (2019). Huang, Yu-Jhe Juang, Jonq Liang, Yu-Hao Wang, Hsin-Yu: Global stability for epidemic models on multiplex networks (2018).īae, Egil Tai, Xue-Cheng Bertozzi, Andrea L.: Simplified energy landscape for modularity using total variation (2018) Berthouze, Luc Miller, Joel C.: Edge-based compartmental modelling of an SIR epidemic on a dual-layer static-dynamic multiplex network with tunable clustering (2018). Li, Zichao Mucha, Peter J.ECG research has been used extensively to diagnose many heart diseases. The ECG is a realistic record of the direction and magnitude of the electrical commotion that is generated by depolarisation and re-polarisation of the atria and ventricles. Most of the clinically useful information on the ECG originates from the intervals and amplitudes defined by their characteristics (characteristic peak and time duration). The improvement of precise and fast methods for the automatic extraction of ECG characteristics is of paramount importance, particularly for the examination of long recordings. In this project we will implement a system that first extracts ECG characteristics and on the basis that we will find the location and amplitude of ECG signal details. ĮCG Feature Extraction plays an important role in the diagnosis of most heart diseases. A cardiac cycle in an ECG signal consists of the P-QRS-T waves. This feature extraction scheme determines the amplitudes and intervals in the ECG signal for further analysis. The value of the amplitudes and the intervals of the segment P-QRS-T determines the functioning of the heart of each human being. Recently, numerous investigations and techniques have been developed to analyse the ECG signal. The proposed schemes were based mainly on fuzzy logic, artificial neural network (ANN), genetic algorithm (GA), vector support machines (SVM) and other signal analysis techniques. All these techniques and algorithms have their advantages and limitations. This paper discusses several techniques and transformations previously proposed in the literature to extract characteristics of an ECG signal. In addition, this paper also provides a comparative study of several methods proposed by researchers in extracting the ECG signal characteristic.